How to: Build a chatbot that generates custom movie scenes using OpenAI
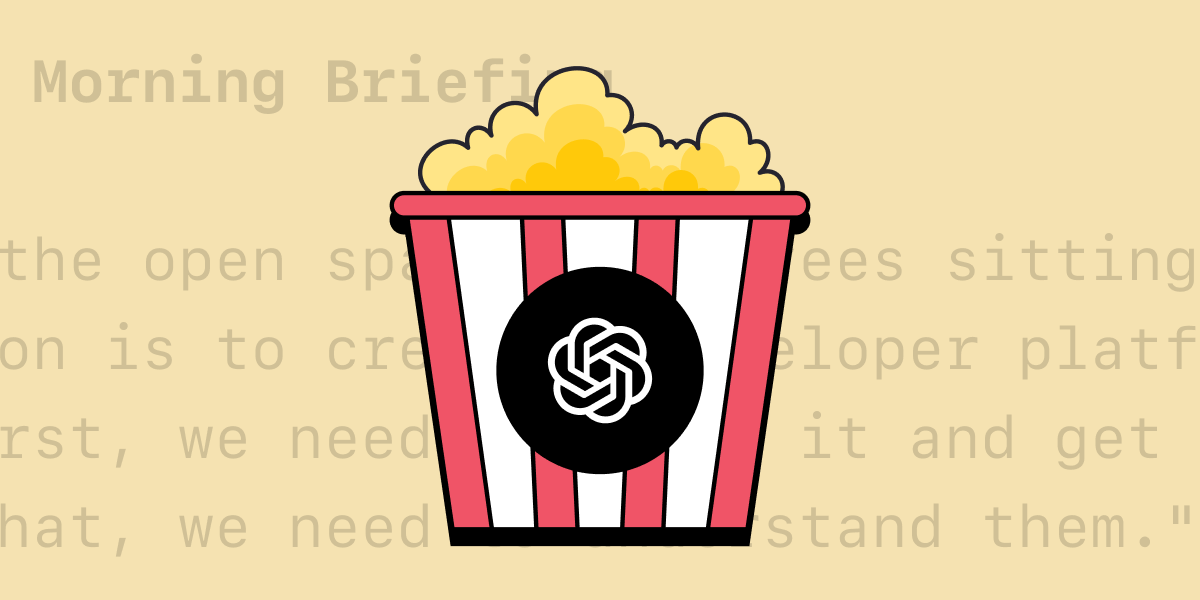
Learn about Gadget's built-in AI features such as the OpenAI connection, vector databases, and cosine similarity search, and use them to build a chatbot that generates custom movie scenes.
You can fork this Gadget project and try it out yourself. After forking, you will still need to run the ingestData global action to ingest some sample data and generate embeddings for the movie quotes. This is detailed in Step 2: Data ingestion.
Create a new Gadget app
Before we get started we need to create a new Gadget app. We can do this at gadget.new. When selecting an app template, make sure you select the AI app template.

Now that we have a new Gadget app, let's start building!
Step 1: Create a movie model
The first thing we need to do is store some movie quotes in our Gadget app. We're going to make use of Gadget's data models, which are similar to tables in a Postgres database, to store this information. To fetch data, we will make use of a global action.
Start by creating a new model in Gadget:
- Click the + button in the DATA MODELS section of the sidebar
- Enter <inline-code>movie<inline-code> as the model's API identifier
Now add some fields to your model. Fields are similar to columns in a database table, and allow you to define what kind of data is stored in your model. For our movie model, we'll add the following fields:
- Click + in the movie model's FIELDS section
- Enter <inline-code>title<inline-code> as the field's API identifier
- Click on the + Add Validations drop-down and select Required to make the <inline-code>title<inline-code> field Required
Adding a Required validation to title means that an error will be thrown if a movie is added without a title. Now let's add a field to store the movie's quotes:
- Click + in the movie model's FIELDS section
- Enter <inline-code>quote<inline-code> as the field's API identifier
- Click on the + Add Validations drop-down and select Required to make the <inline-code>quote<inline-code> field Required
Now we have a place to store movie quotes! This is all the raw required for our app, but we also need a field used to store vector embeddings. Vector embeddings are a way of representing text as a vector of numbers. Gadget has built-in methods to perform similarity searches using vector embeddings - for this app, we'll use these vectors to find similar movie quotes when compared to text entered by users. To learn more about vector embeddings, check out our docs on building AI apps.
- Click + in the movie model's FIELDS section
- Enter <inline-code>embedding<inline-code> as the field's API identifier
- Select <inline-code>vector<inline-code> as the field's type

That is all that we need to store data for our app! Now we need a way to generate embeddings. Luckily, OpenAI has an API that we can use to pass in text and get back a vector embedding.
Step 2: Data ingestion
We need some test data for our app. We're going to make a request to an open data model hosted on Hugging Face. Hugging Face is an AI community and a great resource for AI models, datasets, and great community-provided AI content. We will then use the OpenAI connection to generate embeddings for our movie quotes.
To ingest data into our app, we can make use of a Gadget global action. Unlike model actions, such as the default CRUD actions generated for our movie model, global actions serve as ways to apply updates to more than a single record.
- Click on Global Actions in the sidebar
- Click the + Add Action button or + next to the ACTIONS section title to create a new global action
- Name the action's API Identifier to <inline-code>ingestData<inline-code>
Our OpenAI connection is already set up for us using Gadget-managed credentials. Each team gets $50 in OpenAI credits to use when building in development. To learn more about how to set up your own OpenAI connection, check out our OpenAI connection docs.
With our OpenAI client set up and ready to be used, we can add some code to create embeddings for our movie quotes when they are added to our Gadget database.
- Enter the following code in the generated code file (replace the entire file):
This code:
- uses fetch to pull in a small sample dataset that stores movie quotes hosted on Hugging Face
- loops through the returned data and creates a new movie record for each movie quotes
- uses the OpenAI connection to generate embeddings for each movie quote
- uses your Gadget app's internal API to bulk create movie records with the generated embeddings
Now we can run our global action to ingest the data:
- Click on the Run Action button to open your action in the API Playground
- Run the action
The action will be run and we see a success message once data has been pulled in from the dataset. We can also see the data in our Gadget database by:
- Clicking on the <inline-code>movie<inline-code> model in the sidebar
- Clicking on Data
You should see movie records, complete with title, quote, and embedding data!
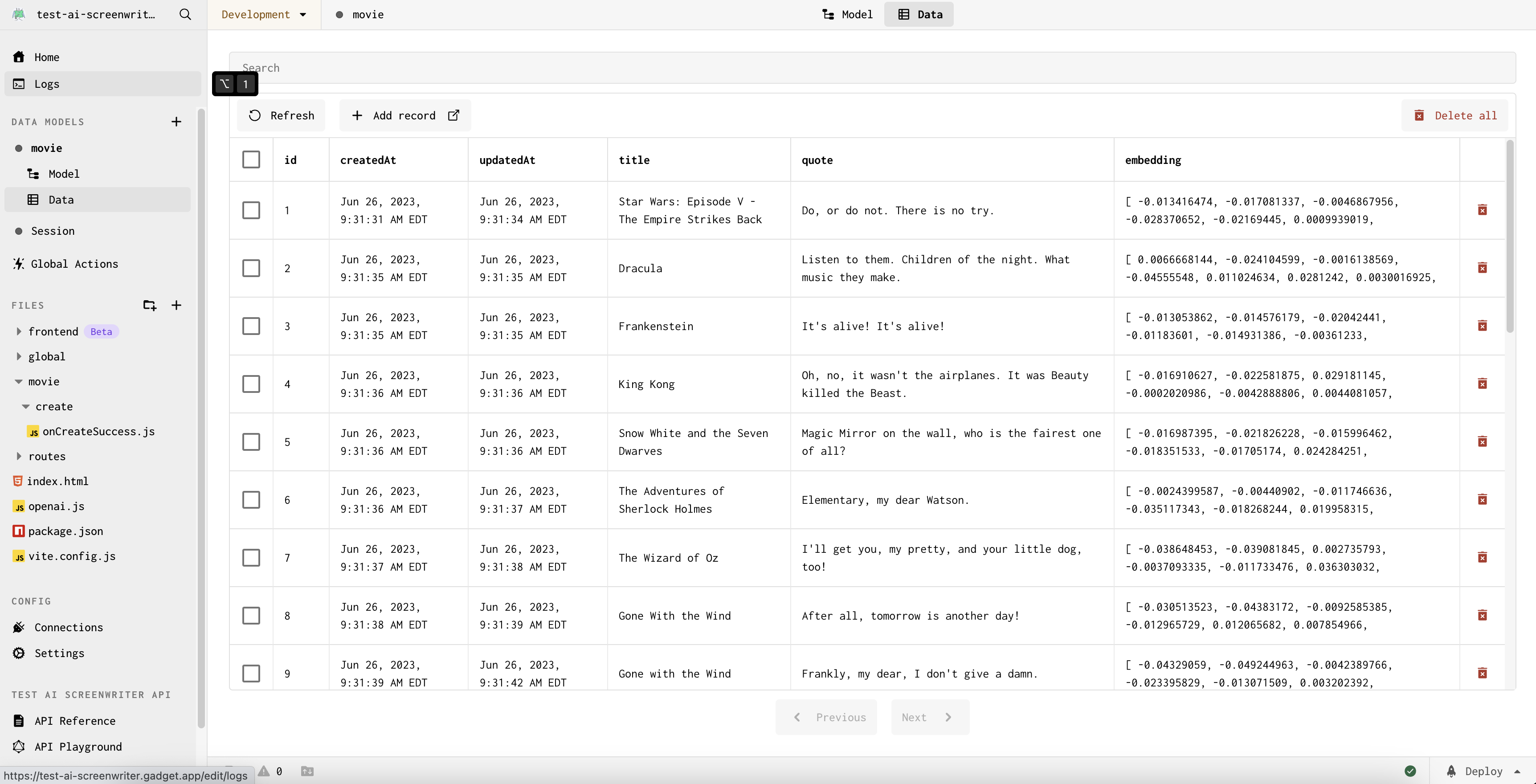
Now that we have data in our database, we are ready to build the user-facing portion of our app.
Step 3: Use a global action to find similar movie quotes
Our app will allow users to enter some text and will find movie quotes that are similar to the entered text using a similarity search on the embeddings. We will use a global action to find the top 4 most similar movie quotes, and then present these movies to the user.
We can create a new global action that we will call from our frontend:
- Click on Global Actions in the sidebar
- Click the + next to the ACTIONS section title to create a new global action
- Name action's API Identifier to <inline-code>findSimilarMovies<inline-code>
- Enter the following code in the generated code file (replace the entire file):
This code creates an embedding from the text passed in by the user and then finds the 4 most similar movies to the entered text. We then remove any duplicate movies and add the movies to the global action's response.
In our global action, we define a custom param at the bottom of the code snippet so the user's quote can be passed in as a string through the <inline-code>params<inline-code> object.
To return values from a global action in Gadget, we need to set the <inline-code>scope.result<inline-code> variable to the value we want to return. In this case, we want to return the movies that are similar to the user's entered text:
Finding similar vectors with cosine similarity
This piece of code, included in the snippet above, is the key to finding similar movies:
Gadget has built-in vector distance sorting which we use to get the most similar vectors to the user's entered text. We use the <inline-code>cosineSimilarityTo<inline-code> operator to find the cosine similarity between the user's entered text and the movie quotes in our database.
Build a UI to display the results
Now let's start building our frontend to call this global action and display the recommended movies to the user.
We're going to use the Chakra UI library to style our frontend. We can install it by running the following commands:
- Open the Gadget command palette with P or Ctrl P
- Type > to enable terminal mode
- Run the following command:
Once installation is complete, we can start building our UI:
- Open the <inline-code>frontend/main.jsx<inline-code> file
- Import the ChakraProvider component at the top of the file:
- Wrap the <inline-code><App /><inline-code> component with the ChakraProvider:
- Replace the contents of <inline-code>frontend/routes/index.jsx<inline-code> with the following code (replace the entire file):
Most of this code is just normal React! The only Gadget-specific code is the <inline-code>useGlobalAction<inline-code> hook, which we use to call our global action. Let's take a closer look at how it works:
We provide our <inline-code>findSimilarMovies<inline-code> global action as input to the <inline-code>useGlobalAction<inline-code> hook. The imported api object, which is your automatically-created Gadget app's API client, is how we can access our Gadget app API from the frontend.
The <inline-code>useGlobalAction<inline-code> hook returns a response object containing:
- <inline-code>data<inline-code> - the data returned from running the action,
- <inline-code>fetching<inline-code> - a boolean that is true when the action is running
- <inline-code>error<inline-code> - an object that details any errors that occurred while running the action
These objects can be used to display returned data, a loading spinner, error banners or messages, when building your UI.
The <inline-code>findSimilar<inline-code> function returned by the hook is what we call to run the action. We pass in the quote as input to the action, and the data returned from the <inline-code>useGlobalAction<inline-code> hook is an array of movies with similar quotes.
Update permissions
By default, newly added actions are not callable by just anyone. We need to grant unauthenticated users permission to call<inline-code> findSimilarMovies<inline-code>.
- Click on Settings in the left sidebar
- Click on Roles & Permissions
- Check the box next to findSimilarMovies under the <inline-code>unauthenticated<inline-code> role
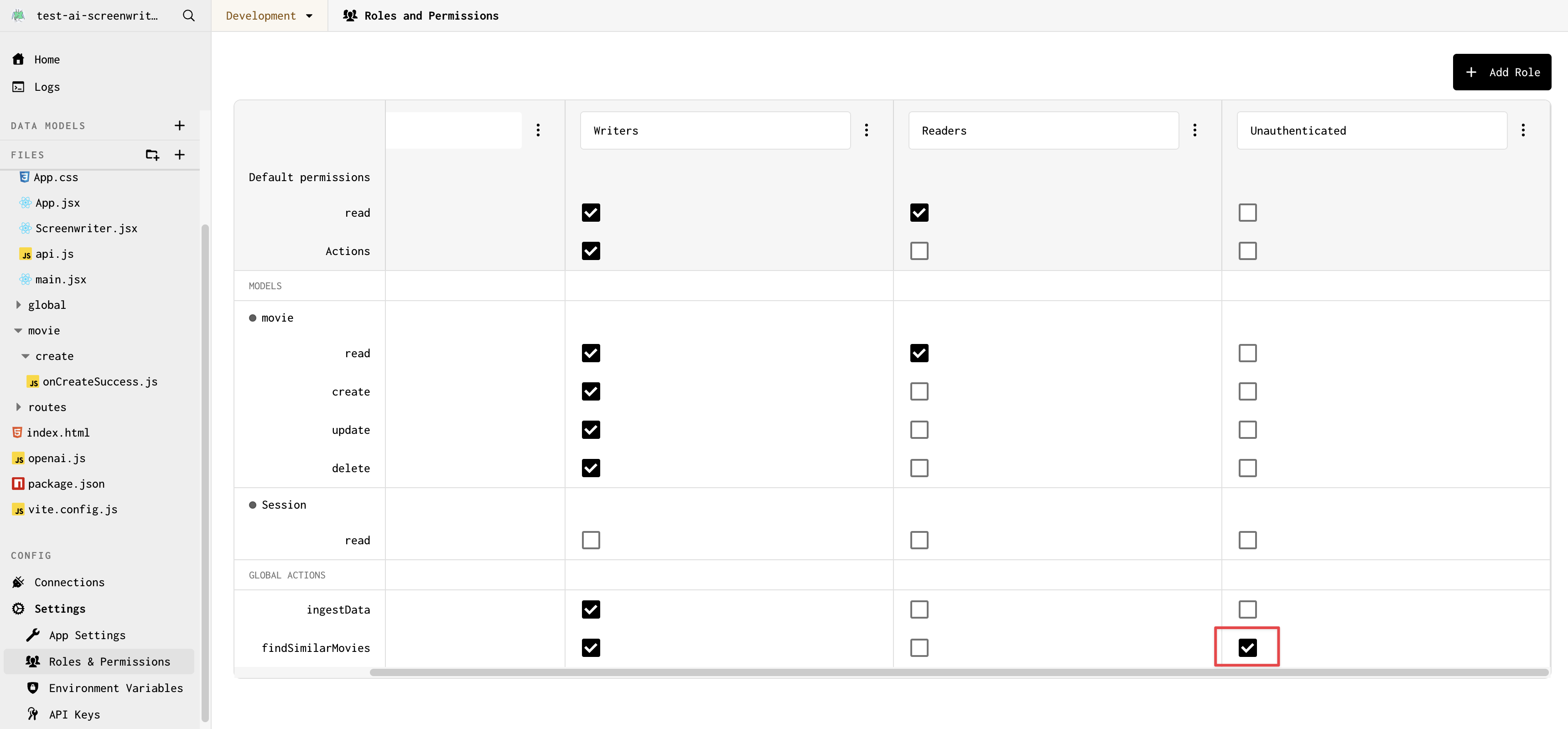
You should now be able to enter a quote and see similar movies! Let's test it out.
Test out the frontend
To view our development app:
- Click on the app name at the top of the left sidebar
- Hover over Go to app and click Development
Try it out! Enter a (fake) movie quote and movies with 'similar' quotes (as decided by our vector similarity search) should be returned.
We're at the last step: let's use OpenAI to generate fake movie scenes using our quote and the selected movie. We can also add some chatbot capabilities to our app so that we can interact with the generated scene.
Step 4: Add a route to generate a scene
Now for the final step in our development process: adding an HTTP route to our Gadget app that will be called by the frontend to generate a scene. We'll use the Vercel AI SDK to make it easy to handle the array of chat responses in our frontend.
Why not use a global action?
We previously used a global action to find similar movies, but we're using a route to generate a scene. You might be asking yourself why? There are two main reasons:
- Global actions do not support streaming responses, and we want to stream the text returned from OpenAI to the frontend
- The openAIResponseStream helper we are using integrates seamlessly with HTTP routes
In general, we suggest you use global actions over HTTP routes whenever possible. But when streaming or integrating with external systems or packages, HTTP routes can be a better choice. To read more about when to use each, see the Actions guide.
- Start by modifying the <inline-code>routes/POST-chat.js<inline-code> HTTP route file in your Gadget app (replace the entire file):
This modifies the sample POST route for our app at /chat.
A custom <inline-code>queryparam<inline-code> is defined in the schema object. This <inline-code>queryparam<inline-code> is used to pass the messages array to the route. The messages array contains the prompt and the generated scene.
We reply using Gadget's <inline-code>openAiResponseStream<inline-code> helper which assists with streaming responses that are returned from the OpenAI connection.
Update the UI to add chatbot functionality
Now that we have defined our route, we can add support to call this route from the frontend. First, install the Vercel AI SDK npm package:
- Open the Gadget command palette with P or Ctrl P
- Type > to enable terminal mode
- Run the following command:
- Once again, paste the following code into <inline-code>frontend/routes/index.jsx<inline-code>:
The <inline-code>useChat<inline-code> hook is imported from Vercel's AI SDK (ai/react) and sends a request to our <inline-code>/chat<inline-code> route when <inline-code>handleSubmit<inline-code> is called.
The <inline-code>useChat<inline-code> hook also provides us with:
- a <inline-code>messages<inline-code> array which we can use to display the chat history
- a <inline-code>setMessages<inline-code> function which we can use to send messages to the chatbot
- a <inline-code>setInput<inline-code> function we use to seed the initial prompt passed into our route
For more details on Vercel's AI SDK, check out the documentation.
None of this added code is Gadget-specific! It is, once again, plain old React, making use of different libraries and packages (Chakra UI and Vercel's AI SDK) to build a simple chatbot.
Test your chatbot
We are done building! Let's test out the AI screenwriter. Enter some text, select a movie, and watch as the AI screenwriter generates a new scene! You can also enter some editor's notes to give the AI screenwriter some feedback.
The final step is deploying to production.
Step 5 (Optional): Deploy toProduction
If you want to deploy a Production version of your app, you can do so in just a couple of clicks!
First, you need to use your own OpenAI API key in the OpenAI connection:
- Click on the Connections tab in the left sidebar
- Click on the OpenAI connection
- Edit the connection and use your API key for the Production environment
Now, deploy your app to Production:
- Click on the Deploy button in the bottom right corner of the Gadget UI
- Click Deploy Changes
That's it! Your app will be built, optimized, and deployed!
You can preview your Production app:
- Click on the app name at the top of the left sidebar
- Hover over Go to app and click Production
Alternatively, you can remove --development from the domain of the window you were using to preview your frontend changes while developing.
Next steps
Congrats! You've built a full-stack web app that makes use of generative AI and vector embeddings! 🎉
In this tutorial, we learned:
- How to create and store vector fields in Gadget
- How to stream chat responses from OpenAI to a Gadget frontend using Vercel's AI SDK
- When to use global actions vs routes in Gadget
This app also contains Gadget's built-in authentication helpers, which we did not cover in this tutorial. If you want to learn more about auth in Gadget, check out the tutorial: Build a blog with authentication
Questions?
If you have any questions, feel free to reach out to us on Discord to ask Gadget employees or the Gadget developer community!